Project Linchpin aims to set Army on sustainable path toward integrating AI into weapons programs
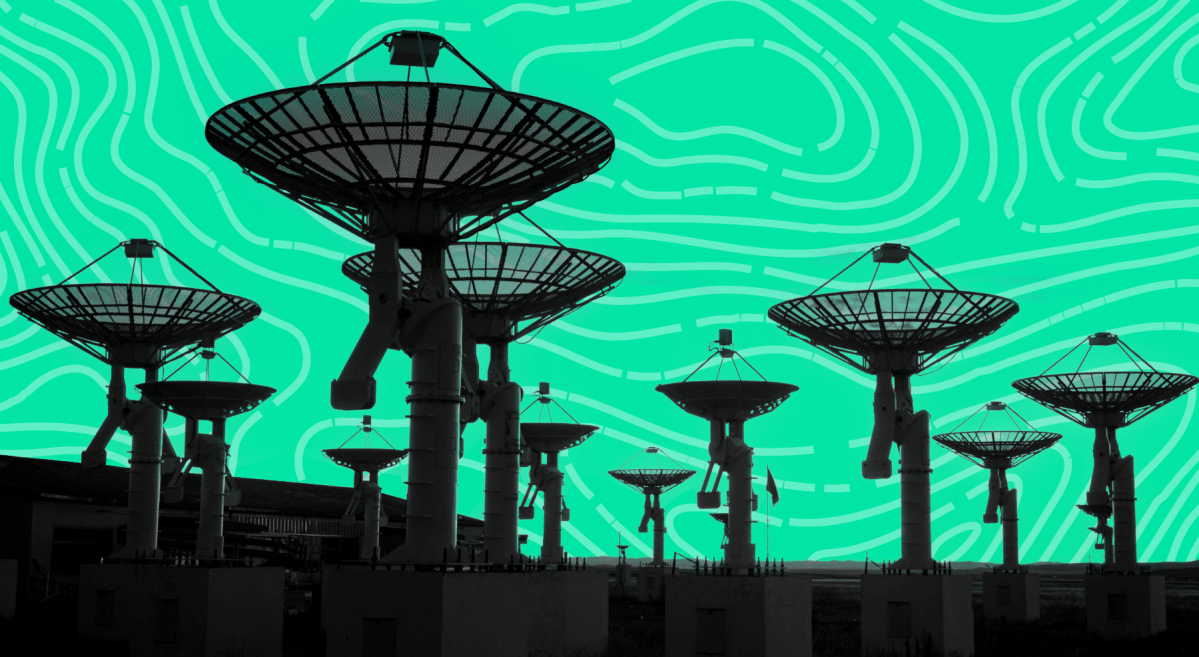
Artificial intelligence isn’t something that just happens at the push of a button. Harnessing the power of advanced computing and analytic capabilities, while in the abstract seems very cool, actually requires a lot of mundane, backend work up front to set the stage for the operational successes military leaders have forecasted.
This is where the Army’s Project Linchpin comes in.
“In the simplest form and concept it’s the way how we are going to deliver AI to the sensor modernization programs,” Bharat Patel, product lead for Project Linchpin at program executive office for intelligence, electronic warfare and sensors, told DefenseScoop in an interview.
Project Linchpin is the Army’s first program of record providing an artificial intelligence operations pipeline. The Army did not respond to multiple requests for information about how many AI programs of record it now has.
“[T]his project takes the standard AI and machine learning operations pipeline from the technology industry and modifies it to perform in a secure government environment while protecting operational data. Project Linchpin offers a secure structure that could be replicated across the Army to deliver AI at scale,” Patel and Maj. Nick Bono, an acquisition officer and systems coordinator for intelligence systems, wrote in a recent article posted to War On The Rocks.
Patel explained to DefenseScoop that while there is nothing novel about an artificial intelligence or machine learning ops pipeline in the commercial world, bringing the concept to government and the military in particular is the challenge.
“Industry has been doing this, we’re going to apply industry best practices, we’re going to leverage commercial technologies to establish this,” he said. “I’m going to decouple all the components within the pipeline and then compete that against industry best of breed, and then bring in industry technology developers to develop models against our sensor modernization programs.”
Patel and Bono wrote that the end state is for Project Linchpin to be a standalone program of record providing a government-owned pipeline that will mitigate risks by breaking up the vertical integration of efforts that have be in place historically.
Project Linchpin will create a disciplined approach that enables continuous integration of machine learning capabilities into Army programs.
“The key really behind that is machine learning — true machine learning — is almost never done. It’s even more rapid than software. Machine learning, you could develop the model, you create the model in a machine learning pipeline, then you put that model in its intended end state,” Patel said. “You put that model in the intended end state, but once the operational data actually hits it, that’s when you see the model performing. That’s when you see what we call model drift — in certain cases, sometimes the model is not performing exactly because there’s a new type of data that comes in. So we have to take that data, bring it back into the operational pipeline, retrain the model and then get that model back into the environment.”
Ultimately, the project seeks to answer a simple question: “How does the Army deliver secure, trusted AI/ML capabilities to AI-enabled systems?” Patel said.
“Project Linchpin recognizes that there are limited resources (funding, technology like high-powered computing, and human capital in the form of AI experts and engineers) for every individual AI-enabled system to manage the process of developing, training, and deploying AI/ML capabilities on their own. Project Linchpin scales that effort to consolidate AI talent and resources in a secure environment. The end state is the reliable delivery of high-performing AI/ML capabilities that users can trust to employ on their systems,” according to Patel.
The effort can trace its roots to Project Maven, an Air Force-led initiative that was created in 2017 to accelerate the adoption of artificial intelligence. It focused initially on processing full-motion video feeds from unmanned aerial systems in the Middle East to support U.S. counterterrorism and counterinsurgency wars. Staff within the program office had been working with the Department of Defense on Project Maven since its inception, leading operational pilots and providing technical oversight.
After the work on Project Maven and coupled with the Army’s push for sensor modernization programs, officials began developing requirements that either implied or specified a need for machine learning, Patel said.
“Going back to my previous knowledge of actually working in this space, in the DOD, and how challenging it was in how complex the different types of tools, technologies [and] industry partners you need to bring in, I made the case to [the program manager] that it was pretty much unaffordable for each PM to go do their own machine learning pipelining,” Patel said.
As a result, he made the case to the PEO last August, briefed it to the principal deputy assistant secretary of the Army for acquisition, logistics and technology last September, and by October, it was announced that the service would be standing up Project Linchpin as a program of record.
Operational outcomes for JADC2
Officials hope Project Linchpin capabilities can ultimately be used across the entire Army.
The main effort to begin with is the Army’s Tactical Intelligence Targeting Access Node (TITAN) program — the service’s next-generation ground system to collect and disseminate sensor data to improve sensor-to-shooter kill chains. It will be a key aspect of not only the service’s so-called deep sensing priority — the ability to discover targets across thousands of miles for long-range fires — but the Pentagon’s new way of war known as Joint All-Domain Command and Control (JADC2).
Patel noted that there’s many components to sensor-to-shooter networks, and an artificial intelligence operations pipeline can help optimize them.
“Our goal is owning that front part of sensor to the shooter is really optimized the ability to identify objects of interest and turn them into — I don’t want to call them targets, but targets of interest,” he said.
For example, an airborne sensor could be sending data to TITAN. As part of that process, TITAN must have algorithms to quickly identify an object and send that information to the shooter. But this capability can eventually be replicated in the aircraft, Patel said, forecasting the eventual goal of perpetuating this pipeline throughout the entire Army.
“Eventually, it’s taking that model and then bringing it into the aircraft. We want to do it for TITAN first and then bring it into the aircraft so that it’s identifying objects at a much more rapid pace,” he said.
Focusing on TITAN was in some ways a strategic decision given that it will receive data from space, air and ground sources. This means officials can build cross-cutting models that span several domains and program offices.
“One of the use cases that we brought to the table was, each PM does not need to build their own machine learning pipeline capability. In addition to that, each PM, even if we have a central pipeline, they don’t need to be in the model development business because this model that was developed for TITAN against [synthetic aperture radar] data, I could just optimize it to work for the aerial side,” Patel said. “This aerial sensor doesn’t need to go out and find its own company to build its own SAR model. I’m just going to tweak and optimize and put it on that system. We’re focusing on TITAN is the right place, because we’re going to have all bunch of sensor data and then we’re just going to optimize and provide all the AIs to the various programs.”
For the time being, Project Linchpin doesn’t appear as its own standalone entity in the Army’s fiscal 2024 budget documents. The only reference to it falls within the TITAN program.
Within TITAN, the documents note that the program will “initiate development and prototyping of Artificial Intelligence/Machine Learning (AI/ML) platforms (i.e., Project Linchpin),” and that a fiscal 2024 funding increase for a portion of the program represents the advancement of artificial intelligence and machine learning integration capabilities through the establishment of Project Linchpin.
Patel and Bono warned, however, that implementation of this pipeline will be critical.
“In most commercial applications the risks of error are just an inconvenience. If Google Maps recommends a faster route that takes you into a traffic jam you might simply be late for your meeting. However, if an intelligence sensor confuses a school bus for a tank — or fails to detect the tank at all — the results could be catastrophic,” they wrote. “No matter how much talk there is about how game-changing AI will be in the future, failing to plan for AI’s ‘back end’ infrastructure is planning to fail.”
In order to get the right data to build off, the program will be relying on the Army’s Office of the Assistant Secretary of the Army for Acquisition, Logistics and Technology (ASAALT) data mesh concept, which aims to essentially standardize the vast quantities of data the service has in order to make it easier to get it to the right decision makers at the right time.
Until that is fleshed out, Patel said officials will be challenged from a data perspective.
“We’re going to be focused on data collection teams in the interim. These data collection teams are going to be responsible for working with each PM to go … grab some data and bring that into our repository,” he said.
To train the algorithms properly, they might only need a fraction of the hours and hours of full-motion video, for example, because that small tidbit is really important to the algorithms’ development. The rest isn’t. To do that would be extremely manual.
But when ASAALT’s model comes online, that should relive some of that burden, Patel said.
“We are going to be challenged, but we have a path to address some of that as it relates to the sensor part of it. But then once ASAALT comes online with the data fabric and data mesh, I think it’s going to start being a little bit cleaner,” he explained.
Non-traditional contractors
When it comes to industry support, Patel said officials are looking primarily at non-traditional contractors and small businesses.
“We’re constantly going out to industry looking for who has the best technology for model training, who has the best technologies for model development? That’s right for the non-traditionals and small businesses,” he said.
The most recent request for information went out to industry in March, and the team is planning to use an upcoming Technical Exchange Meeting in May to provide more information.
Moreover, the Project Linchpin team briefed the effort to Army senior leadership in April with additional discussions planned going forward.
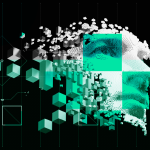
This story was featured in DefenseScoop Special Report: Operational AI in the U.S. Military — A DefenseScoop Special Report